Panel Recap: Incrementality from a Data Science Perspective
January 21, 2021
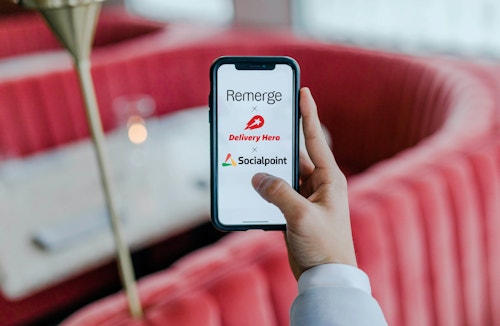
Did you miss our first data science focused online event at the App Promotion Summit’s WFH edition? Don’t fret - we’ve captured everything for you in this summary and recap of key points. This 60-minute video covers the different themes, questions, and problems around incrementality measurement and performance marketing.
Key Points and Video Recording
Learn about the following:
- Our guests personal stories in data science
- Working with different stakeholders on the complexities of incrementality measurement
- When to start implementing incrementality
- How to run incrementality tests
- How to validate results and communicate uncertainties
Hosted by our Product Specialist Federica Stiscia, our panel of data scientists included:
- Johannes Haupt (Senior Data Scientist at Remerge)
- Alicia Horsch (Marketing Data Scientist at Socialpoint)
- Yue Meng (Data Scientist at Delivery Hero)
Recap of Key Points
While the concept of incrementality is no longer new, its application to advertising is relatively new. When running these types of experiments, the current problem our industry faces is the lack of standardization or written rules.
While there are many questions that are answered by incrementality, what do data scientists want to answer with it? When did it also become relevant to measure marketing campaign success with incrementality?
For Socialpoint, it started because of retargeting campaigns. They wanted to know what the return on their retargeting efforts were. From their research, they learned that incrementality is the new market standard of measuring uplift.
Delivery Hero started with incrementality four years ago, also wanting to know about their marketing campaign ROI, especially with retargeting as they continually launched products in new markets. They also needed marketing campaigns to maintain exposure and grow revenue in markets where they’re established. The scientific tests from incrementality help them understand if their spending is/was truly meaningful.
Stakeholder management
While the industry works towards standardizing incrementality, what are the questions and complex topics that data scientists usually have to explain to stakeholders?
Data scientists are usually confronted with business-facing questions: “Can we make the control group smaller because we don't want to lose users if they were exposed?”, “Does the calculation from our partners make sense?” Sometimes it also involves explaining some concepts such as if the P-value is 0.49 and the difference to significance is a point estimate, why the campaign can’t be paused just yet.
For companies that are relatively new to incrementality, it’s about finding the best setup: finding a methodology and the people they want to reach. Would they be people who churned months ago or people who are still active? Discussions also involve why it would be better to look at test vs control instead of control vs exposed. While the latter is more appealing to marketers, it is an incorrect method because of selection bias.
On the other hand, experienced clients know what incrementality is, and are convinced that it is relevant, but the questions are around technical details and how to interpret the results. For example, statistical significance - “What does it mean exactly and how do I use that?”. A lot are also related to statistics in general - how it works with attribution and what it means if the attributed numbers are different.
Validating results
How do you confirm and validate the significance of the results and how do you communicate the degree of uncertainty?
In the early stages, make sure that the group split is alright before going into the test. Next, double check that all the procedures are correct - check with the partner that the way of splitting control and test groups make sense. With stakeholders, define conversion from the start. For example, checking the front page, adding to cart, acquisition or order, are all different types of conversions. While they form a funnel, each stage could be a metric to check. Forecast on one KPI that you really want to check, hold the others for later.
When it comes to uncertainties, using the Bayesian approach helps to make sure that the result is really stable. For other uncertainties, only confirm when it applies only on a certain range. For example, if the campaign group is 20-30yo and has significant results, the same results might not hold if when applying the same campaign to the 40-50yo group.
Incrementality Testing
How do you conduct incrementality measurement?
The concept is straightforward but the practise requires deep thinking on what to measure. In retargeting, you can address the users multiple times - make sure to randomize on a user-level so that they stay on the test or control group.
Also be careful about the control group as it is the baseline conversion rate. With lift tests, the historical control group conversion rate can be a reference when another test makes sense. For example, if you target the same market with a similar population, the conversion of this test and the historical test shouldn’t be so different. However, this might only apply to stable markets. For new markets, the acquisition curve might be higher, so a test from a few months ago might have different conversion rates.
Finally, working with different partners might be tricky, so consider taking a methodology that allows you to compare.
Expanding Knowledge
Which research papers or articles do our panelists follow?
Alicia relies on practical content such as blog posts and articles from practitioners like the Remerge blog or third-parties like AppsFlyer. Yue and Johannes agree, adding that reading the raw code helps to see how other data scientists approach the same question (github).
Additional sources include the “Recent Developments of Econometrics on Program Evaluation” by Imbens and Woolridge is quite readable, and the Online Causal Seminar showcase of the best researches worldwide.
Questions from the audience
POST-IDFA
How do you adapt your incrementality models and assumptions for a post-IDFA world?
One way is to find something similar without the user ID is to use technical features for randomization. For example, test each city and compare the traffic (a method previously used in TV). In digital we have more options to do this - find a solution to that problem first, by replicating the randomization on some level.
Statistical Significance
Any Tips on how to reduce statistical insignificance and how to have smaller confidence intervals?
Theoretically-speaking, collect a small population size as possible and the distribution will become narrower and get a more significant test. Realistically, the easiest way is to run a longer experiment: get more users or have a more balanced split to maximize what you want to get out of a week’s worth of data, by going for a 50/50 split. Then from there consider shifting from the Intent-to-treat to Ghost bids methodology where you can filter out the exposed group.
Additionally, the Bayesian approach can give the conclusion that both groups do not make any difference.
Also, understand that from a business perspective, significance is not always the answer - one campaign could give 1-3% and another 2-4% but if the target was 10%, it doesn’t matter which campaigns are significant compared to the other.
Long Term Effect
The incremental outcomes are usually shorter. How do you look at the long term effect and how do you calculate or predict long term incremental LTV and payback windows?
Have an always-on test. Set up a holdout group which never gets ads and run the test for at least six months then compare the different conversion rates of both groups. It depends what you’re looking for - for installs, the effects are immediate whereas LTV purchases take more time to take place. Ultimately you can achieve this through a longer experimental duration and a longer control group.